Azure Databricks vs HDInsight: A Detailed Business Software Analysis
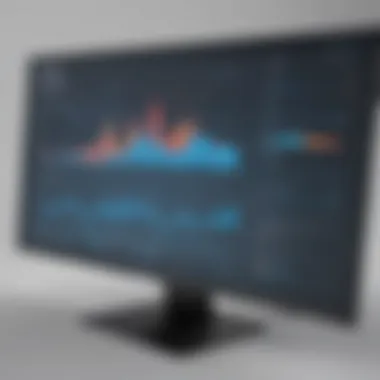
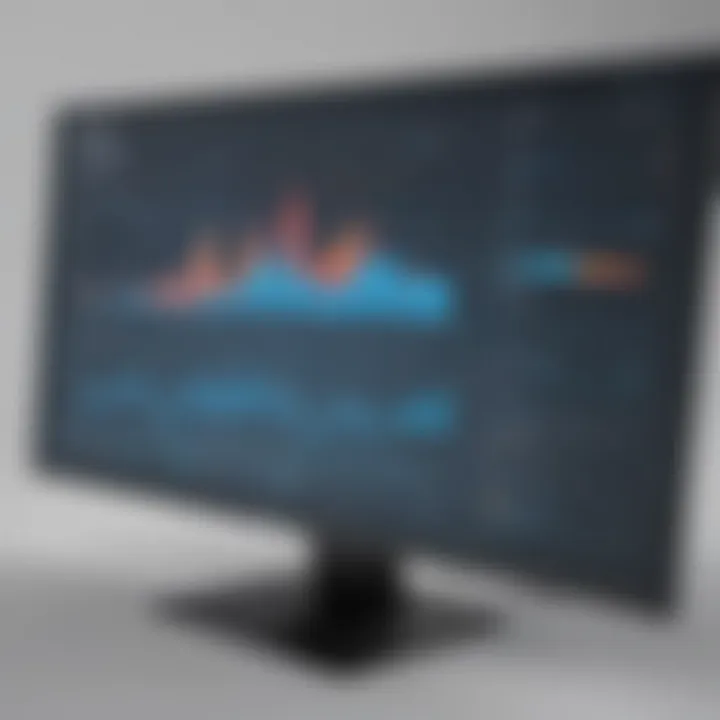
Software Overview
Azure Databricks and HDInsight are two stalwart players in the realm of big data and analytics platforms, each offering a unique array of features and functionalities. Azure Databricks, a collaborative Apache Spark-based analytics service, provides a unified approach to data analytics, machine learning, and AI capabilities. In contrast, HDInsight, a cloud-based big data analytics service, focuses on open-source frameworks such as Apache Hadoop and Apache Spark to handle large-scale data processing tasks efficiently. When considering software options, evaluating the key features, functionalities, pricing plans, and available subscriptions becomes pivotal for businesses seeking optimal solutions.
Introduction to the Software
Azure Databricks, backed by Microsoft Azure, empowers users with a seamless environment for data engineering, collaborative data science, and real-time analytics. Its integration with Azure services enhances scalability and flexibility. On the other hand, HDInsight leverages the power of open-source technologies for processing vast datasets efficiently, providing users with a versatile platform for diverse big data applications.
Key Features and Functionalities
Azure Databricks offers interactive workspace, automated cluster management, and collaboration tools to streamline data workflows. Its machine learning capabilities and integration with Azure Machine Learning service enable advanced analytics. Conversely, HDInsight stands out with its compatibility with a wide range of open-source frameworks, Hadoop and Spark clusters, and support for multiple programming languages, catering to varying business needs.
Pricing Plans and Available Subscriptions
Azure Databricks pricing is based on a combination of virtual machines and storage resources, allowing users to scale resources as needed. HDInsight follows a pay-as-you-go model, offering flexibility in resource allocation and cost management. Understanding the pricing structures and available subscription options is essential for businesses to align software expenses with budgetary constraints and operational requirements.
Performance and Reliability
Considering the performance and reliability aspects of Azure Databricks and HDInsight sheds light on their operational efficiency and value proposition within the big data landscape. The speed and efficiency of data processing, uptime statistics, and integration capabilities with external tools play crucial roles in determining the software's operational robustness.
Security and Compliance
Protecting sensitive data and ensuring regulatory compliance are paramount for businesses leveraging big data platforms like Azure Databricks and HDInsight. Evaluating data encryption measures, security protocols, adherence to industry regulations, and backup and disaster recovery solutions is essential to safeguard critical business information and mitigate potential risks.
Introduction
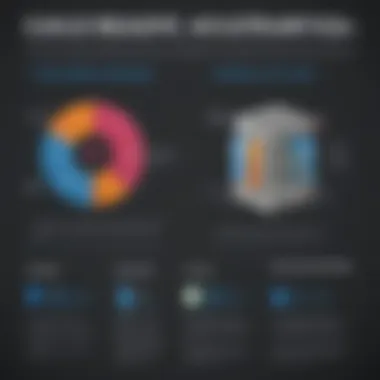
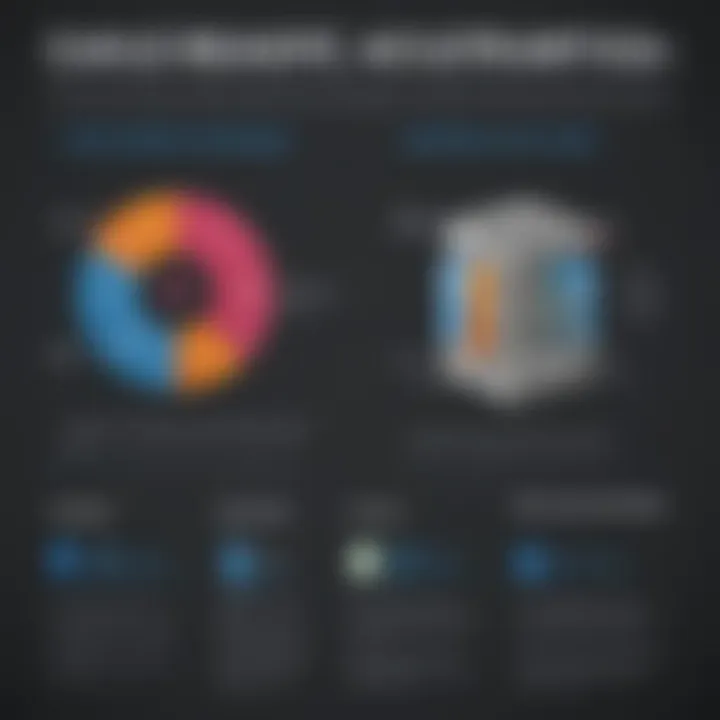
In the realm of big data and analytics, Azure Databricks and HDInsight stand as prominent platforms commanding attention from small to medium-sized businesses, entrepreneurs, and IT professionals. Understanding the nuances and disparities between these two solutions is not just important but crucial for informed decision-making. This article embarks on a journey of thorough comparison, dissecting the features, scalability, performance, pricing models, and practical applications of Azure Databricks and HDInsight. By illuminating the intricacies of these platforms, this guide aims to equip readers with the knowledge necessary to navigate the complex landscape of business software choices effectively.
Overview of Azure Databricks and HDInsight
As we immerse ourselves in the competitive arena of big data and analytics, Azure Databricks and HDInsight emerge as titans in the field, each offering distinct advantages and functionalities. Azure Databricks combines the power of Apache Spark with Microsoft's Azure cloud services, providing a unified analytics platform that fosters collaborative and streamlined data-driven decision-making. In contrast, HDInsight, a fully managed cloud service by Microsoft Azure, focuses on facilitating the deployment and management of open-source analytical clusters, catering to organizations seeking scalable and cost-effective big data solutions.
Significance of Choosing the Right Big Data and Analytics Platform
The significance of selecting an apt big data and analytics platform cannot be overstated in today's fast-paced business landscape. An ideal platform not only empowers businesses to extract valuable insights from vast data pools but also streamlines operations, enhances competitiveness, and drives innovation. Choosing between Azure Databricks and HDInsight entails deliberating on factors such as scalability, performance, integration capabilities, and pricing structures, all of which play pivotal roles in shaping the efficiency and effectiveness of data-driven initiatives.
Purpose of the Comparison
The main purpose of juxtaposing Azure Databricks and HDInsight lies in offering a comprehensive evaluation that aids decision-makers in aligning their software needs with the distinctive offerings of these platforms. By delving deep into the features, performance metrics, scalability options, integration capabilities, and real-world applications of Azure Databricks and HDInsight, this comparison serves as a compass, guiding businesses towards selecting the optimal big data and analytics solution. Understanding the intricacies of these platforms equips users with the knowledge required to harness the full potential of big data, fueling growth and innovation in the digital age.
Feature Comparison
In the realm of big data and analytics platforms, the feature comparison carries paramount significance as it delineates the core functionalities that distinguish Azure Databricks from HDInsight. By scrutinizing features such as scalability, performance, and integration capabilities, businesses can discern the nuances that could impact their operations. Analyzing these elements empowers decision-makers to select the platform that aligns most closely with their specific requirements, paving the way for optimal utilization of resources and enhanced productivity.
Scalability
Scalability in Azure Databricks
When delving into the intricacies of scalability within Azure Databricks, one encounters a robust framework designed to accommodate varying workloads seamlessly. The key characteristic of this platform lies in its ability to scale effortlessly, catering to the evolving demands of data processing. Azure Databricks stands out as a preferred choice due to its streamlined scalability features, ensuring that businesses can expand their analytical capabilities without encountering performance bottlenecks. The unique scalability feature of Azure Databricks lies in its auto-scaling functionality, which dynamically adjusts resources based on workload, optimizing efficiency. While this aspect presents numerous advantages by enhancing flexibility and cost-efficiency, it may also pose challenges in terms of resource management and cost prediction, necessitating vigilant monitoring and optimization strategies.
Scalability in HDInsight
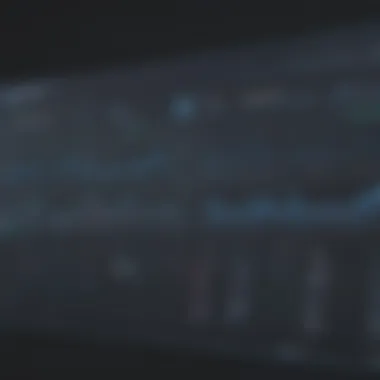
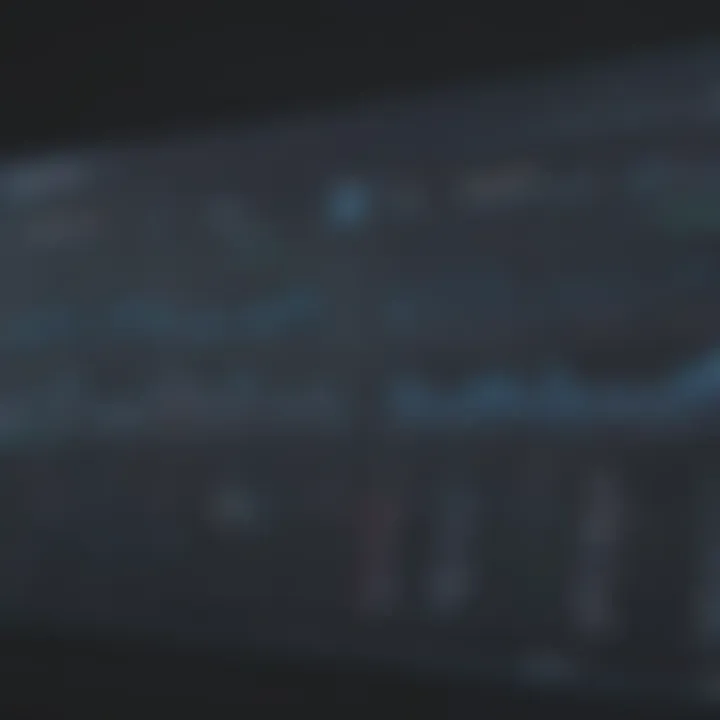
In contrast, the scalability aspect of HDInsight revolves around its capacity to ramp up operations swiftly to meet escalating computational demands. The defining characteristic of scalability in HDInsight highlights its ability to handle data-intensive tasks effectively, positioning it as an attractive option for organizations with substantial data processing requisites. HDInsight's unique feature in scalability lies in its seamless integration with other Azure services, enabling seamless scalability across interconnected systems. While this integration offers unparalleled advantages in terms of interoperability and performance optimization, it may introduce complexities in managing interconnected infrastructures and dependencies, warranting a strategic approach to scalability implementation.
Performance
Performance Metrics in Azure Databricks
The discussion on performance metrics within Azure Databricks sheds light on the platform's operational efficiency and data processing speed. A key characteristic of performance metrics in Azure Databricks is its ability to execute complex computations rapidly, bolstering decision-making processes for users. The standout feature in performance metrics for Azure Databricks is its optimization for parallel processing, which accelerates data processing tasks significantly. While this feature enhances operational speed and productivity, it may also entail challenges in fine-tuning parallel processing settings for optimal performance, necessitating expertise in leveraging this functionality effectively.
Performance Metrics in HDInsight
Conversely, when evaluating performance metrics in HDInsight, the focus shifts towards gauging the platform's capability to deliver high-speed data analytics and processing functionalities. A key characteristic of HDInsight's performance metrics lies in its comprehensive monitoring and diagnostic tools, empowering users to analyze performance bottlenecks and optimize system efficiency. The unique feature in performance metrics for HDInsight is its integration with popular analytical tools and frameworks, enhancing interoperability and data processing agility. While this integration drives operational efficiency and analytical capabilities, it may introduce challenges related to compatibility and customization, necessitating thorough evaluation to harness its full potential.
Integration Capabilities
Integration Features in Azure Databricks
Efficient integration capabilities play a pivotal role in determining the interoperability and connectivity of Azure Databricks within existing business ecosystems. The key characteristic of integration features in Azure Databricks lies in its seamless assimilation with various data sources and tools, fostering a cohesive data environment. Azure Databricks' unique feature in integration lies in its native integration with Azure services, simplifying data workflows and enhancing data accessibility across diverse platforms. While this native integration streamlines data management and enhances operational efficiency, it may present challenges concerning data security and governance, underscoring the need for robust data protection measures and compliance protocols.
Integration Features in HDInsight
On the other hand, the integration features of HDInsight underscore its versatile connectivity options and compatibility with a myriad of data processing technologies. The critical characteristic of integration features in HDInsight is its extensibility through open-source frameworks, allowing users to leverage a wide array of tools for data analysis and processing. HDInsight's unique feature in integration capabilities lies in its support for hybrid cloud environments, facilitating seamless data movement between on-premises and cloud-based systems. While this hybrid cloud support offers unmatched versatility and scalability, it may introduce complexities in data synchronization and management, necessitating strategic planning and execution to ensure smooth integration and operational continuity.
Use Cases
When contemplating the selection of a big data and analytics platform, understanding the possible applications is paramount. In the realm of business software decision-making, use cases play a pivotal role in illuminating the practical scenarios in which Azure Databricks and HDInsight can be deployed. The significance of use cases lies in their ability to showcase real-world scenarios, shedding light on how these platforms can address specific business needs and challenges. By examining concrete examples of their usage, potential users can gain valuable insights into how Azure Databricks and HDInsight can be leveraged to optimize processes, enhance efficiency, and extract meaningful insights from vast quantities of data. Scrutinizing diverse use cases can help stakeholders assess the suitability of each platform for their unique requirements, guiding them towards a well-informed decision.
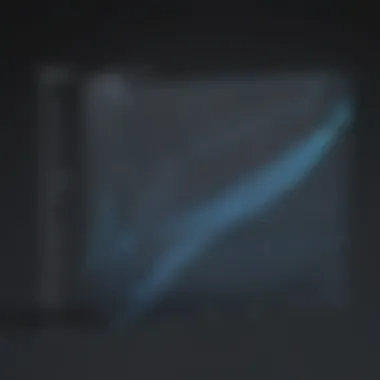
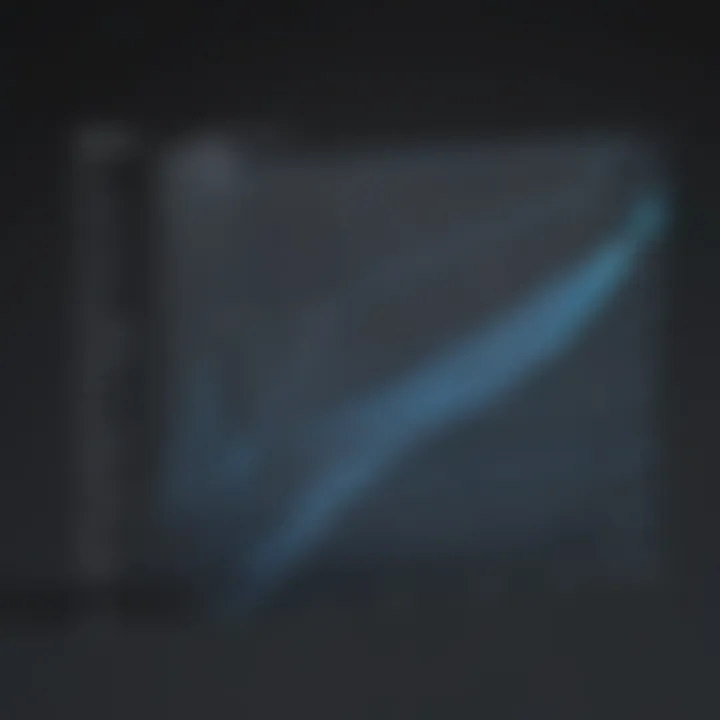
Real-World Applications of Azure Databricks
Within the realm of Azure Databricks, real-world applications abound across various industries and domains. One prominent application of this platform is in financial services, where it is utilized for fraud detection, risk analysis, and personalized customer experiences. The healthcare sector also benefits from Azure Databricks, leveraging its capabilities for predictive analytics, patient monitoring, and medical research. Moreover, e-commerce businesses leverage this platform for customer segmentation, recommendation engines, and supply chain optimization. By harnessing the power of Azure Databricks, organizations can streamline operations, gain predictive insights, and drive innovation across multiple fronts.
Real-World Applications of HDInsight
Contrary to Azure Databricks, HDInsight finds its niche in different real-world applications that cater to distinct requirements. In the realm of Io T, HDInsight is instrumental for processing and analyzing vast amounts of sensor data for predictive maintenance and operational efficiency. In the energy sector, this platform is utilized for optimizing drilling operations, predictive maintenance of machinery, and energy grid management. Additionally, retail enterprises rely on HDInsight for demand forecasting, inventory optimization, and personalized marketing initiatives. The versatility of HDInsight's applications highlights its adaptability across various sectors, showcasing its prowess in addressing specific industry challenges and driving data-driven decision-making.
Pricing Models
Pricing models play a pivotal role in the decision-making process for businesses when evaluating big data and analytics platforms such as Azure Databricks and HDInsight. Understanding the pricing structures of these platforms is crucial for budgeting and cost management. Businesses need to consider various elements when assessing pricing models, including subscription plans, usage-based pricing, additional costs for extra features, and any potential discounts or promotions available. By comprehensively examining the pricing models of Azure Databricks and HDInsight, businesses can make well-informed decisions that align with their financial objectives and operational requirements.
Cost Structures in Azure Databricks
Delving into the cost structures of Azure Databricks provides insights into how organizations can anticipate and manage expenses related to deploying this platform. Azure Databricks offers flexible pricing options, including pay-as-you-go models and enterprise agreements. Understanding the intricacies of cost components such as compute costs, storage fees, and data transfer expenses is essential for optimizing cost efficiency. By analyzing the cost structures of Azure Databricks, businesses can tailor their usage to align with their budget constraints while maximizing the value derived from this advanced analytics solution.
Cost Structures in HDInsight
Exploring the cost structures of HDInsight unveils the financial implications associated with leveraging this big data platform. HDInsight provides pricing models based on factors such as virtual machines, storage usage, and data processing capabilities. By assessing the cost structures of HDInsight, businesses can gain visibility into the total cost of ownership and identify opportunities for cost optimization. Understanding the cost breakdown of HDInsight enables organizations to make data-driven decisions that balance performance requirements with budgetary considerations for sustainable long-term usage.
Conclusion
In navigating the vast landscape of big data and analytics platforms, the topic of the conclusion plays a pivotal role in synthesizing the intricate details discussed throughout this article. It serves as the cornerstone where key insights are distilled and form the bedrock for strategic decision-making processes. The meticulous exploration of Azure Databricks and HDInsight unveils a plethora of nuances and considerations that are indispensable for business software decision-making. By dissecting the features, scalability, performance metrics, integration capabilities, real-world applications, and pricing models of both platforms, this comparison equips small and medium-sized businesses, entrepreneurs, and IT professionals with the necessary arsenal to make informed choices pertaining to their software needs. The significance of the conclusion lies in its ability to offer a comprehensive overview, consolidating the nuances explored throughout the article into actionable points that direct the reader towards thoughtful and data-driven decisions.
Key Takeaways
As we dissect the intricacies of Azure Databricks and HDInsight, several key takeaways emerge, shedding light on crucial aspects that demand attention in the realm of big data and analytics platforms. Firstly, it becomes apparent that scalability is a pivotal factor, with Azure Databricks exhibiting formidable scalability features that cater to varying business requirements. On the other hand, HDInsight boasts robust performance metrics, showcasing its proficiency in handling complex data processing tasks. The integration capabilities of both platforms present unique strengths, with Azure Databricks excelling in seamless integration features and HDInsight offering a diverse array of integration possibilities. Real-world applications highlight the adaptability of these platforms across different industries, underscoring their versatility and relevance in today's data-centric business landscape. Lastly, the pricing models of Azure Databricks and HDInsight showcase diverse cost structures, providing businesses with options tailored to their budgetary considerations.
Final Recommendations
In light of the exhaustive comparison between Azure Databricks and HDInsight, final recommendations can be synthesized to guide business software decision-making effectively. Small to medium-sized businesses seeking robust scalability and agile performance may find Azure Databricks to be a compelling choice, given its advanced scalability features and proficient performance metrics. Alternatively, entrepreneurs and IT professionals focusing on integration capabilities and diverse use cases may lean towards HDInsight for its versatility and broad range of integration features. Understanding the distinctive pricing models of both platforms is crucial, enabling businesses to align software needs with budgetary constraints effectively. Ultimately, the choice between Azure Databricks and HDInsight boils down to a nuanced evaluation of specific business requirements, considering factors such as scalability, performance, integration, real-world applications, and cost structures to make a well-informed decision that propels business operations towards heightened efficiency and success.
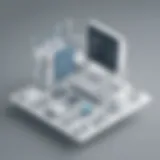
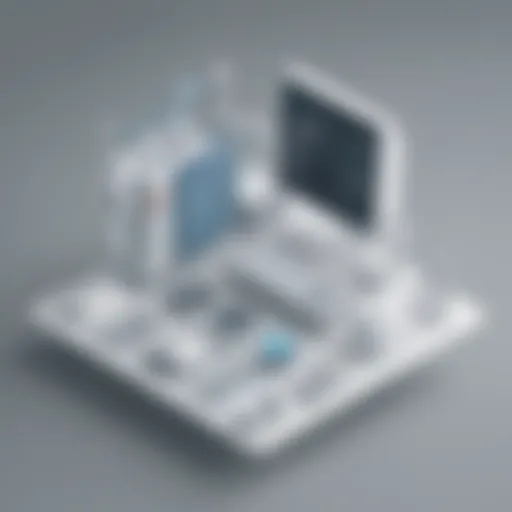