Exploring the Latest Insights and Trends in Big Data Statistics for 2021
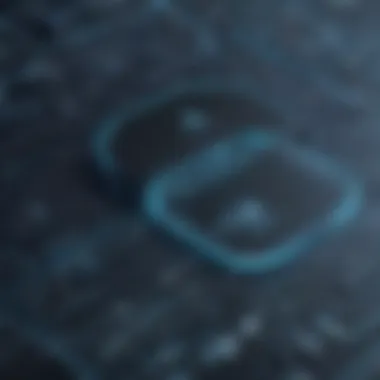
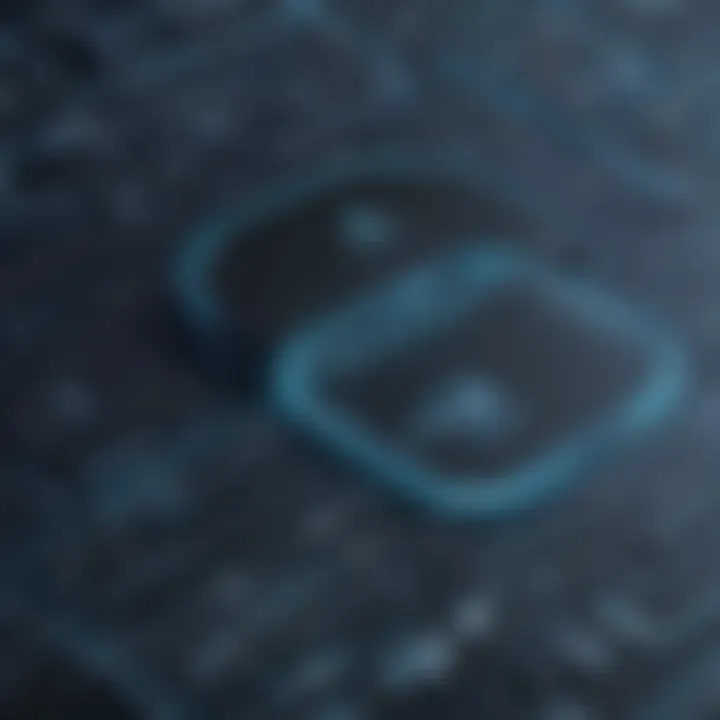
Software Overview
In the realm of big data statistics for 2021, having the right software is crucial for businesses seeking to harness the power of data. This section delves into an insightful overview of the software landscape, providing a detailed look at the key features, functionalities, and pricing plans available to businesses.
User Experience
A seamless user experience is vital when working with big data statistics. This subsection explores the user interface, navigational elements, and ease of use of the software. Additionally, it discusses the learning curve involved and the customer support options offered, ensuring businesses can effectively leverage these tools.
Performance and Reliability
When dealing with large datasets, performance and reliability are paramount. This part delves into the speed and efficiency of the software, along with statistics on uptime and downtime. Moreover, it examines the integration capabilities with other tools, enabling businesses to streamline their operations.
Security and Compliance
Protecting data integrity is a top priority in the world of big data. This section evaluates the data encryption methods and security protocols employed by the software, ensuring compliance with industry regulations. Backup and disaster recovery measures are also scrutinized, bolstering data protection for businesses in the digital landscape.
Introduction to Big Data
In the realm of data analytics, the concept of 'Big Data' plays a pivotal role in shaping business strategies and operations. This section serves as a cornerstone for understanding the sheer magnitude and complexity of data in the modern landscape. Big Data encapsulates vast and diverse datasets that traditional data management tools may struggle to handle efficiently. By delving into the world of Big Data, businesses can harness valuable insights, drive informed decision-making, and gain a competitive edge in their respective industries.
Defining Big Data
Volume of Data
The volume of data refers to the sheer quantity of information generated and collected by organizations. This massive influx of data presents both challenges and opportunities in the context of Big Data analytics. The key characteristic of volume lies in its exponential growth, with businesses accumulating terabytes, petabytes, or even exabytes of data. This abundance of data provides a rich source for analysis and pattern recognition, enabling companies to uncover hidden trends, correlations, and customer behaviors. However, handling such vast volumes of data requires robust infrastructure and advanced analytical tools, which can be a costly investment for organizations. Balancing the benefits of extensive data insights with the complexities of storage and processing remains a central consideration for leveraging volume effectively in the realm of Big Data.
Velocity of Data
The velocity of data pertains to the speed at which data is generated, processed, and analyzed in real-time. In today's fast-paced digital landscape, businesses are inundated with data streams from various sources such as social media, Io T devices, and online transactions. The key characteristic of velocity lies in the rapid influx of data that demands immediate attention and action. Real-time analytics have become essential for extracting actionable insights, detecting anomalies, and responding proactively to market trends. Despite the advantages of timely data processing, managing high-velocity data streams can strain existing IT infrastructures and necessitate scalable solutions for capturing, storing, and analyzing data effectively.
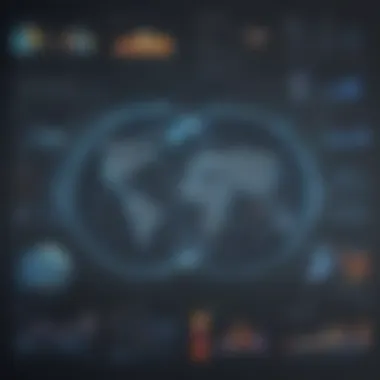
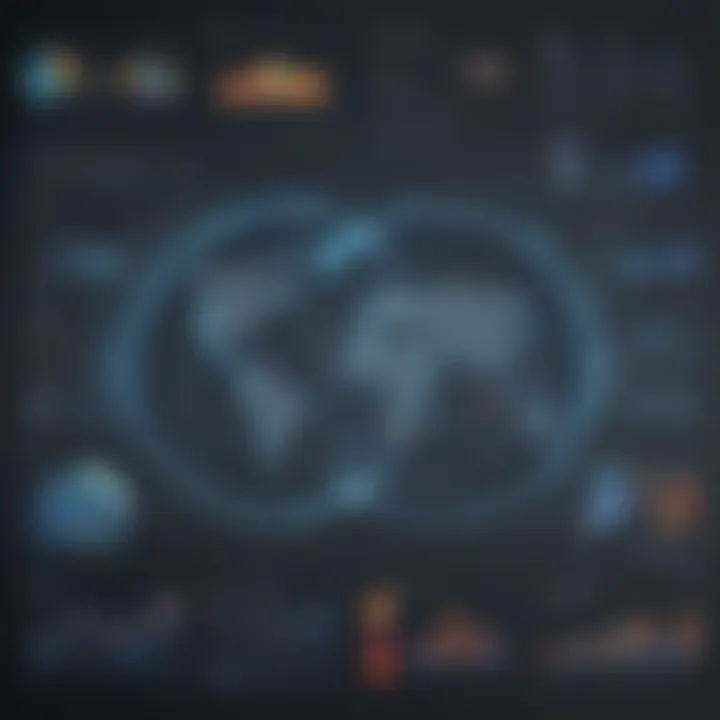
Variety of Data
The variety of data encompasses the diverse forms and formats in which data is generated and stored across platforms and systems. Big Data sources include structured data from databases, semi-structured data from JSON or XML files, and unstructured data from social media posts, images, and videos. The key characteristic of variety lies in the heterogeneous nature of data types that pose challenges for integration, analysis, and interpretation. By embracing diverse data sources, organizations can gain a holistic view of their operations, customer interactions, and market trends. However, managing varied data formats requires flexible data pipelines, interoperable systems, and advanced analytics techniques to derive meaningful insights from this data mosaic.
Significance of Big Data Analytics
Data-Driven Decision Making
Data-driven decision-making empowers organizations to base their strategic choices on empirical evidence, statistical analysis, and predictive modeling. By leveraging Big Data analytics, businesses can move beyond intuition and gut feelings to prioritize data-backed insights that enhance operational efficiency and revenue growth. The key characteristic of data-driven decision-making lies in its objectivity and scalability, enabling organizations to derive actionable intelligence from vast datasets. However, the over-reliance on data without human judgment can lead to analysis paralysis or overlooking qualitative factors that influence decision outcomes. Balancing quantitative analysis with qualitative judgment remains essential for realizing the full potential of data-driven decision-making in a dynamic business environment.
Predictive Analytics
Predictive analytics involves forecasting future trends, behaviors, and outcomes based on historical data patterns and advanced algorithms. By applying machine learning models and statistical techniques to Big Data sets, organizations can anticipate customer preferences, market fluctuations, and potential risks. The key characteristic of predictive analytics lies in its forward-looking focus that empowers businesses to preempt challenges, capitalize on opportunities, and optimize resource allocation. However, predictive models are inherently probabilistic and subject to data biases, requiring continuous refinement and validation to enhance predictive accuracy. Maintaining a balance between predictive insights and real-time feedback loops is crucial for maximizing the value of predictive analytics in a competitive business landscape.
Personalization
Personalization entails tailoring products, services, and customer experiences to individual preferences, interests, and behaviors. Through Big Data analytics, organizations can segment their customer base, deliver targeted offers, and customize interactions to enhance engagement and loyalty. The key characteristic of personalization lies in its focus on customer-centricity and relationship building that drives long-term value for both businesses and consumers. However, navigating privacy concerns, data security regulations, and ethical considerations is essential when crafting personalized experiences. Finding the right balance between hyper-personalization and consumer trust is key to fostering sustainable relationships and brand loyalty in the era of data-driven marketing.
Key Trends in Big Data
In the ever-evolving landscape of big data, staying abreast of key trends is paramount for businesses seeking to remain competitive and innovative in 2021. The infusion of Artificial Intelligence (AI) into big data processes has been a focal point, revolutionizing how data is processed and analyzed. One of the prominent trends within AI integration is the surge in Machine Learning Applications, enabling systems to learn from data patterns and make informed decisions autonomously. Machine learning offers the benefit of enhancing predictive capabilities and streamlining data analysis procedures. Moreover, Natural Language Processing (NLP) has gained traction for its ability to decipher and derive meaning from human language data, facilitating more effective communication between man and machine. This trend towards AI-driven big data analytics marks a significant shift towards more efficient and insightful data processing methods.
Artificial Intelligence Integration
Machine Learning Applications
When delving into the realm of Machine Learning Applications, one cannot overlook its pivotal role in revolutionizing data analytics. Machine learning, a subset of AI, empowers systems to automatically learn and improve from experience without being explicitly programmed. This facet contributes to the overarching goal of optimizing data analytics processes and honing decision-making based on data-driven insights. The key characteristic of Machine Learning Applications lies in their ability to recognize complex patterns within vast datasets, enabling businesses to extrapolate valuable information efficiently. This functionality proves beneficial for this article as it enhances the understanding of data trends and aids in predictive modeling. Despite its advantages, one must also acknowledge the possible limitations such as the requirement for substantial initial data and potential algorithm biases.
Natural Language Processing
Natural Language Processing (NLP) stands out as a cornerstone of AI integration within big data analytics. NLP focuses on enabling machines to comprehend, interpret, and generate human language, bridging the gap between data processing and human interaction. The key characteristic of NLP lies in its capacity to extract meaning from textual data, facilitating sentiment analysis, language translation, and speech recognition. This feature proves to be advantageous in this article as it allows for contextual understanding of unstructured data, thereby enhancing the depth of data analysis and interpretation. Despite its benefits, challenges such as semantic ambiguity and linguistic complexities pose potential hurdles in accurate data processing and interpretation.
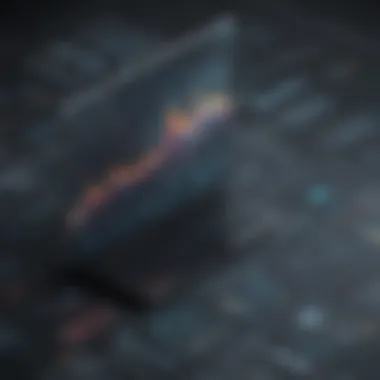
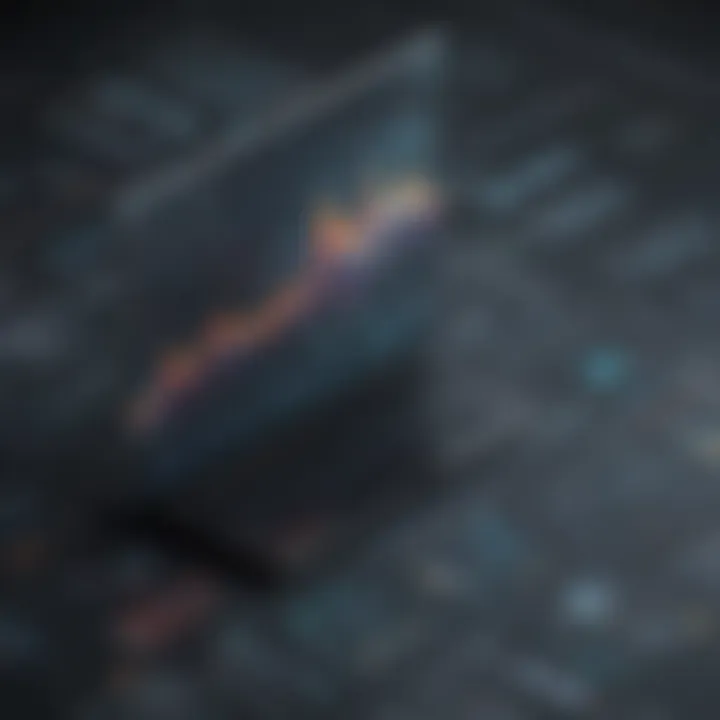
Edge Computing
Decentralized Data Processing
In the digital age, Edge Computing emerges as a revolutionary trend in big data management, reshaping the traditional centralized data processing paradigm. Decentralized Data Processing, a core component of Edge Computing, involves moving data processing closer to the data source rather than in a centralized data center. This strategy aims to reduce latency, enhance processing speed, and improve data security. The unique feature of Decentralized Data Processing lies in its ability to execute computational tasks at the edge of the network, enabling real-time data analysis and decision-making. This approach proves beneficial for this article by fostering quicker data processing and enabling seamless integration of Io T devices and sensors at the network edge. However, challenges such as network connectivity issues and security vulnerabilities must be carefully addressed in the implementation of Decentralized Data Processing.
Real-time Insights
Real-time Insights represent a pivotal aspect of Edge Computing, enabling businesses to derive instant and actionable information from data streams. Real-time data processing stands out for its ability to provide immediate insights into dynamic datasets, facilitating prompt decision-making and agile business operations. The key characteristic of Real-time Insights lies in its capacity to process and analyze data in near-real-time, ensuring that businesses can respond swiftly to changing market trends and consumer behaviors. This functionality is advantageous for this article as it allows for the exploration of real-time analytics applications and the generation of instant insights for business optimization. Nevertheless, challenges such as data accuracy and scalability need to be addressed to leverage the full potential of Real-time Insights.
Data Security and Privacy
GDPR Compliance
In today's data-centric environment, adhering to data protection regulations such as the General Data Protection Regulation (GDPR) is paramount to ensure the privacy and security of sensitive information. GDPR Compliance entails implementing measures to safeguard personal data, enhance transparency in data processing practices, and uphold individual data rights. The key characteristic of GDPR Compliance lies in its focus on data minimization, purpose limitation, and accountability, aiming to foster greater trust between businesses and individuals. This compliance framework proves beneficial for this article by emphasizing the significance of ethical data handling and promoting data privacy best practices. However, the complexities of GDPR requirements and potential penalties for non-compliance underscore the importance of robust data governance structures and compliance mechanisms.
Cybersecurity Measures
Amid the digital age's proliferation, Cybersecurity Measures have emerged as an indispensable component of big data operations to mitigate cyber threats and safeguard sensitive data assets. Cybersecurity Measures encompass a range of strategies, protocols, and technologies aimed at preventing unauthorized access, data breaches, and malicious activities. The key characteristic of Cybersecurity Measures lies in their role in fortifying data infrastructure, identifying vulnerabilities, and responding to security incidents promptly. This aspect proves beneficial for this article by highlighting the critical importance of integrating cybersecurity practices within big data frameworks to mitigate risks effectively. Nonetheless, the evolving nature of cyber threats and the sophistication of cyber-attacks necessitate continuous vigilance and adaptive cybersecurity strategies to uphold data integrity and protect against potential breaches.
Applications of Big Data
Big Data has become a critical component for businesses in 2021, revolutionizing how organizations operate and make decisions. The utilization of Big Data in businesses has shown substantial benefits and has become imperative for staying competitive in the market. By leveraging data analytics, businesses can extract valuable insights that drive strategic decision-making and improve operational efficiency. The applications of Big Data span across various sectors, showcasing its versatility and potential impact on business growth and innovation.
Marketing and Customer Insights
Targeted Advertising
Targeted Advertising is a strategic approach that focuses on tailoring marketing efforts to specific demographics or customer segments. This personalized advertising technique allows businesses to customize their campaigns based on consumer behavior, preferences, and demographics. Utilizing Big Data in targeted advertising enables companies to optimize their marketing strategies, increase customer engagement, and enhance conversion rates. The ability to target the right audience with precision has proven to be a cost-effective and efficient marketing tactic, driving better ROI and customer satisfaction.
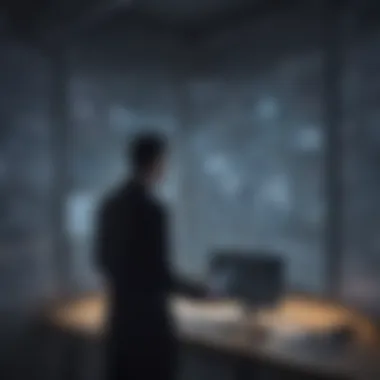
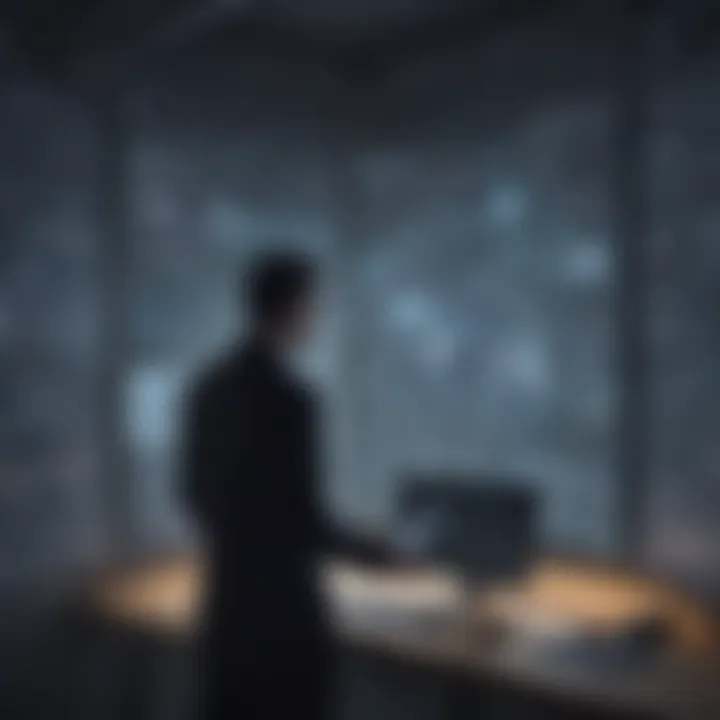
Behavioral Analysis
Behavioral analysis in Big Data involves the evaluation of consumer behavior patterns and interactions with products or services. By analyzing customer behavior data, businesses can gain valuable insights into consumer preferences, decision-making processes, and trends. Behavioral analysis enables companies to personalize customer experiences, improve product offerings, and strengthen customer relationships. While offering deep insights, behavioral analysis also presents challenges such as data privacy concerns and the need for robust data security measures.
Healthcare and Predictive Analytics
Disease Diagnosis
Disease diagnosis through Big Data analytics involves the use of advanced algorithms to interpret medical data and identify potential health conditions. This application of Big Data enables healthcare professionals to diagnose illnesses accurately, predict disease outcomes, and devise suitable treatment plans. By leveraging predictive analytics in disease diagnosis, healthcare providers can enhance patient care, optimize treatment procedures, and improve overall healthcare outcomes. However, the reliance on predictive analytics for disease diagnosis also raises ethical considerations surrounding patient privacy and data confidentiality.
Treatment Optimization
Treatment optimization using Big Data entails analyzing patient data to develop personalized treatment plans and enhance medical procedures. By leveraging data insights, healthcare providers can customize treatments based on individual characteristics, medical history, and predictive analytics. Treatment optimization through Big Data empowers healthcare professionals to deliver personalized care, improve treatment effectiveness, and reduce medical errors. Despite its advantages, treatment optimization faces challenges such as data integration complexities, regulatory compliance, and the need for skilled data scientists and healthcare professionals.
Challenges and Opportunities
Data Quality Management
Data Governance
Data governance plays a pivotal role in ensuring that data within an organization is reliable, secure, and compliant with regulations. It encompasses the policies, processes, and controls necessary to manage data assets effectively. In the context of this article, data governance is highlighted as a critical component of data quality management. Its key characteristic lies in establishing clear ownership and accountability for data-related decisions and actions. By implementing robust data governance practices, businesses can enhance data integrity, mitigate risks, and improve decision-making processes. However, a potential disadvantage of stringent data governance frameworks is the possibility of hampering agility in data-driven initiatives.
Quality Assurance
Quality assurance focuses on maintaining and improving the quality of data throughout its lifecycle. It involves determining quality standards, performing quality checks, and implementing corrective measures where necessary. Within the scope of this article, quality assurance is depicted as a fundamental aspect of data quality management. Its key characteristic revolves around continuous monitoring and evaluation of data quality metrics to ensure data reliability and accuracy. By emphasizing quality assurance practices, organizations can enhance data usability, credibility, and stakeholder trust. Nevertheless, a challenge of quality assurance lies in the resource-intensive nature of conducting comprehensive quality assessments, which may impact operational efficiency.
Skill Shortage in Data Science
The shortage of skilled professionals in the field of data science presents a critical challenge for organizations aiming to leverage big data for strategic decision-making and innovation. Addressing this skill gap requires a strategic focus on developing talent through training programs and implementing effective recruitment strategies. This section explores the nuanced components of skill shortage, focusing on the significance of training and recruitment initiatives in overcoming this obstacle and nurturing a data-literate workforce.
Training Programs
Training programs are instrumental in equipping individuals with the knowledge and skills required to excel in data-related roles. They offer structured learning experiences encompassing theoretical concepts, practical applications, and hands-on training. In the context of this article, training programs are highlighted as a key solution to address the skill shortage in data science. Their unique feature lies in tailored curriculum design that aligns with industry demands and emerging technologies. By investing in comprehensive training programs, organizations can upskill their workforce, foster innovation, and stay abreast of evolving data trends. However, a challenge associated with training programs is the evolving nature of technology, which necessitates continuous updates to training modules and materials.
Recruitment Strategies
Recruitment strategies play a pivotal role in attracting, selecting, and retaining top talent in the field of data science. They encompass the processes and techniques employed to source candidates, assess their skills, and onboard them effectively. In the context of this article, recruitment strategies are portrayed as vital mechanisms for addressing the skill shortage in data science. Their unique feature lies in leveraging diverse talent pools, including non-traditional sources, to expand the recruitment reach and access potential candidates with varied skill sets. By implementing targeted recruitment strategies, organizations can build a robust data science team, drive innovation, and foster a culture of data-driven decision-making. Nonetheless, a challenge associated with recruitment strategies is the competitive landscape for data science talent, which requires innovative approaches to attract and retain skilled professionals.