Uncovering Diverse Options Beyond BigQuery for Data Warehouse Needs
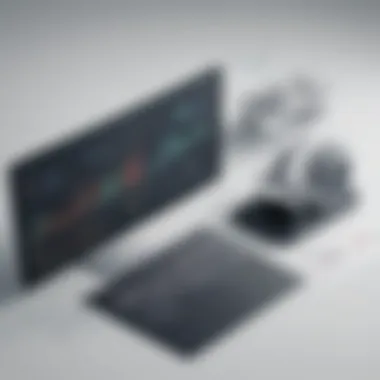
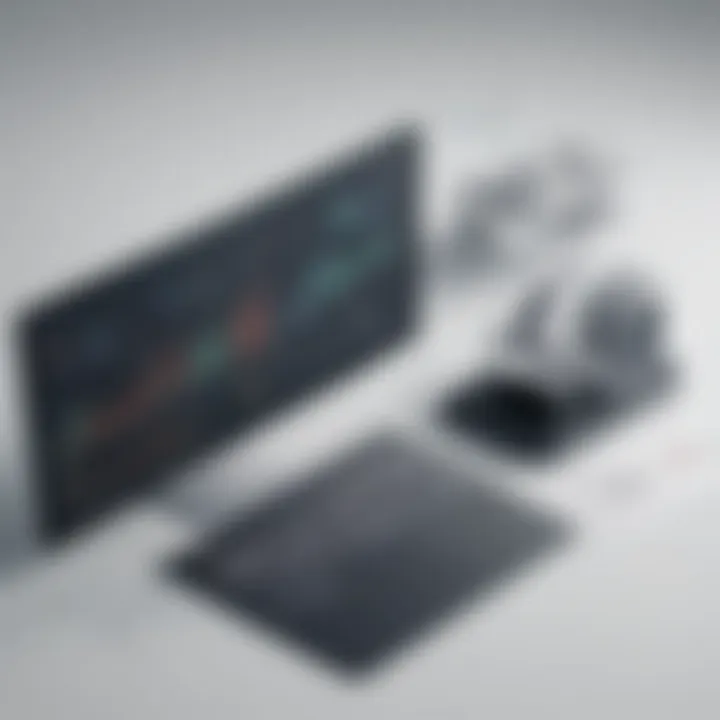
Software Overview
Snowflake's pricing plans and available subscriptions are structured to cater to the specific needs of different users. With options for on-demand pricing based on usage, as well as more tailored enterprise-level packages, Snowflake ensures accessibility while accommodating scalability requirements and budget constraints.
User Experience
In terms of user experience, Snowflake shines with its intuitive user interface and navigational elements. The platform's design prioritizes clarity and simplicity, reducing the learning curve for new users and enhancing overall usability. Navigating through data streams and analytics tools is made effortless, thanks to the well-crafted user experience that Snowflake offers.
Moreover, Snowflake's robust customer support options contribute to its user-friendly nature. The platform provides reliable customer support services, ensuring that users receive timely assistance and guidance whenever they encounter challenges or have inquiries. This dedication to customer satisfaction further enhances the overall user experience of utilizing Snowflake.
Performance and Reliability
Snowflake excels in terms of performance and reliability, boasting impressive speed and efficiency in processing data queries and operations. The platform's architecture is optimized to deliver swift results, even when handling complex analytical tasks and large datasets. Additionally, Snowflake's uptime statistics demonstrate a consistently reliable service, minimizing downtime and ensuring seamless data availability for users.
Integration capabilities are another strong suit of Snowflake, enabling seamless interoperability with various third-party tools and data sources. The platform's compatibility and flexibility make it a versatile choice for businesses looking to integrate their existing data ecosystems with a powerful data warehouse solution like Snowflake.
Security and Compliance
When it comes to security and compliance, Snowflake prioritizes data encryption and stringent security protocols. The platform implements robust measures to safeguard data privacy and integrity, offering users peace of mind regarding the confidentiality of their sensitive information. Snowflake also maintains compliance with industry regulations, ensuring that data handling practices align with established standards and requirements.
Backup and disaster recovery measures are integral components of Snowflake's security framework. The platform incorporates reliable backup solutions and disaster recovery protocols to mitigate risks and ensure data continuity in unforeseen circumstances. By prioritizing security and compliance, Snowflake establishes itself as a trustworthy and secure data warehouse option for businesses seeking reliable data storage and analytics capabilities.
Introduction
Understanding the Need for Alternatives
Challenges with BigQuery
One of the primary challenges with BigQuery lies in its scalability limitations when handling massive datasets. While BigQuery is renowned for its ease of use and swift query processing, it may encounter performance bottlenecks as the volume of data grows exponentially. This limitation can hinder businesses' ability to derive insights quickly from extensive datasets, prompting the exploration of alternative solutions that offer enhanced scalability.
Exploring Diverse Requirements
In the quest for comprehensive data storage and analytical solutions, businesses often have diverse requirements that go beyond what BigQuery can offer. These requirements could range from specialized data processing needs to unique cost considerations. Exploring these diverse requirements is vital to ensuring that the chosen alternative aligns with the specific demands of the business, thereby optimizing data management processes.
Criteria for Evaluation
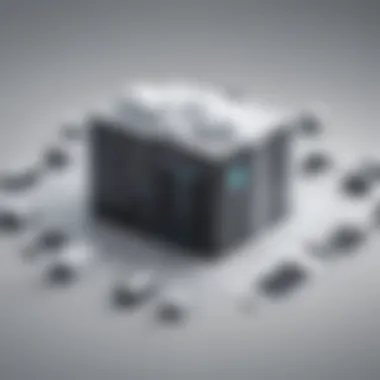
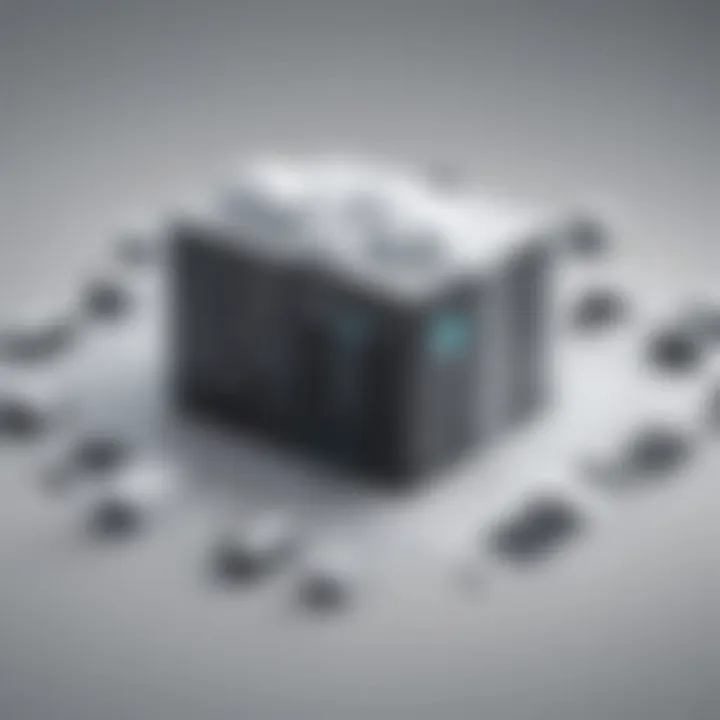
Performance Metrics
Performance metrics play a pivotal role in evaluating the efficacy of data warehousing solutions. Factors such as query speed, concurrency handling, and overall system responsiveness are critical in determining the performance capabilities of a platform. By scrutinizing performance metrics, businesses can make informed decisions regarding the adoption of alternative solutions that offer superior performance benefits.
Cost Considerations
Cost considerations form a fundamental aspect of selecting an alternative to BigQuery. While BigQuery is renowned for its cost-effectiveness in certain scenarios, businesses must explore alternative solutions that offer competitive pricing models without compromising performance. Balancing cost considerations with performance metrics is essential in ensuring a cost-effective yet efficient data warehousing solution.
Scalability Needs
Scalability needs encompass the ability of a data warehousing solution to expand and handle growing data volumes seamlessly. While BigQuery provides decent scalability features, businesses with exceptionally high data growth rates may seek alternatives that offer limitless scalability options. Evaluating scalability needs is critical in future-proofing data management strategies and preventing scalability constraints.
Importance of Choosing the Right Alternative
Aligning with Business Goals
Alignment with business goals is paramount when selecting an alternative to BigQuery. The chosen solution should complement the organization's broader objectives, whether it's enhancing operational efficiency or fostering data-driven decision-making. By aligning with business goals, businesses can ensure that the selected alternative contributes positively to overall strategic initiatives.
Ensuring Data Security
Data security remains a top priority for businesses traversing the data warehousing landscape. The alternative solution chosen should prioritize robust data security measures to safeguard sensitive information from unauthorized access or breaches. Ensuring data security not only instills customer trust but also mitigates potential risks associated with data vulnerabilities.
Alternatives to Consider
When delving into the realm of alternatives to BigQuery, it is vital to understand the importance of this exploration. In a landscape where data storage and analytics are fundamental for businesses of all scales, the quest for viable alternatives holds significant relevance. Small to medium-sized enterprises, entrepreneurs, IT professionals, and decision-makers are continually seeking innovative solutions to enhance their operations. By examining various alternatives to BigQuery, these entities can unlock new possibilities and efficiencies in managing their data.
Snowflake
Architecture Overview
In scrutinizing Snowflake's architecture, one cannot overlook its intricate design that sets it apart in the realm of data warehousing. The distributed nature of Snowflake's architecture allows for seamless scalability and performance optimization, catering to the evolving needs of modern enterprises. By decoupling storage and compute resources, Snowflake ensures cost efficiency without compromising on processing power. This unique architectural model not only enhances flexibility but also simplifies management, making Snowflake a prudent choice for those looking to elevate their data infrastructure.
Key Features
Delving into Snowflake's key features sheds light on its prowess in the realm of data warehousing. Snowflake's ability to support diverse data workloads, ranging from traditional SQL queries to massive analytical tasks, underscores its versatility. The platform's automatic scaling capabilities enable users to handle varying workloads effortlessly, ensuring optimal performance at all times. Additionally, Snowflake's built-in data sharing functionality facilitates collaboration and data monetization, adding another layer of value to its offerings. While these features pave the way for enhanced operational efficiency, users must navigate potential complexities in data governance and access control.
Amazon Redshift
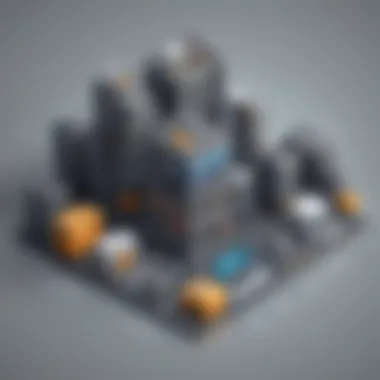
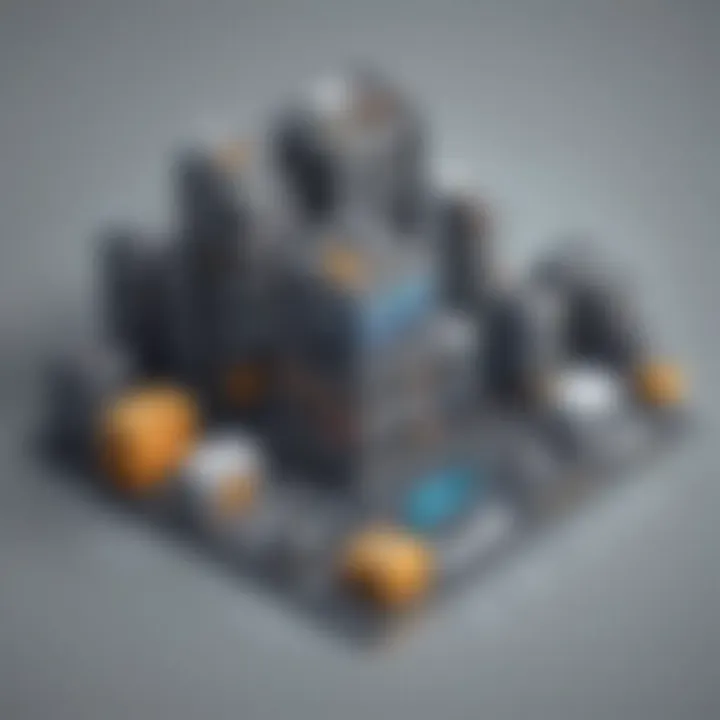
Comparative Analysis
Examining Amazon Redshift from a comparative standpoint reveals its strengths and weaknesses in the data warehousing arena. The platform's columnar storage and parallel processing capabilities position it as a frontrunner in terms of query performance and scalability. Amazon Redshift's integration with other Amazon Web Services amplifies its appeal by offering a holistic cloud ecosystem for data management. However, users must be attuned to potential cost implications associated with data transfer and storage on the platform, warranting a strategic approach to resource utilization.
Integration Capabilities
Assessing Amazon Redshift's integration capabilities unveils its seamless adaptability within diverse IT environments. The platform's compatibility with various data sources and visualization tools streamlines the data pipeline, fostering a cohesive analytics ecosystem. Amazon Redshift's support for open-source technologies further enhances its interoperability, empowering users to leverage existing frameworks efficiently. Yet, users may encounter constraints in complex data transformation workflows, necessitating tailored optimization strategies for sustained performance.
Google Cloud Datastore
Use Cases
Exploring the practical applications of Google Cloud Datastore illuminates its utility across various scenarios. The platform's NoSQL database functionality caters to agile development environments, enabling rapid iterations and flexibility in data schema architecture. Google Cloud Datastore's robust replication and backup features instill confidence in data durability and disaster recovery, critical for mission-critical applications. Nonetheless, users must navigate the platform's limitations in handling complex querying tasks and relational data structures, ensuring alignment with specific use case requirements.
Limitations
Unpacking the limitations of Google Cloud Datastore provides insights into its operational boundaries and constraints. Despite its strengths in horizontal scalability and ease of use, the platform may face challenges in managing high-volume transactional workloads efficiently. Google Cloud Datastore's eventual consistency model poses considerations for applications reliant on real-time data accuracy, necessitating strategic data modeling and synchronization approaches. While the platform offers robust support for web and mobile applications, users must evaluate its suitability for workloads demanding stringent data consistency and integrity.
Apache Cassandra
Scalability Features
Surveying Apache Cassandra's scalability features underscores its robust architecture tailored for high-throughput environments. The platform's decentralized peer-to-peer design empowers seamless horizontal scaling, supporting massive data volumes and concurrent operations. Apache Cassandra's decentralized architecture minimizes single points of failure, bolstering fault tolerance and resilience in distributed setups. Nonetheless, users must navigate the intricacies of data modeling and configuration to harness Cassandra's full potential, ensuring optimal performance without compromising data integrity.
Querying Capabilities
Exploring Apache Cassandra's querying capabilities sheds light on its distinctive approach to data retrieval and manipulation. The platform's support for CQL (Cassandra Query Language) streamlines query execution, offering a familiar SQL-like interface for users. Apache Cassandra's tunable consistency levels enable users to fine-tune read and write operations based on application requirements, balancing performance with data accuracy. While these querying capabilities empower users with flexibility and performance optimization, they necessitate a nuanced understanding of data distribution and replication strategies for efficient query processing.
Microsoft Azure SQL Data Warehouse
Performance Insights
Delving into Microsoft Azure SQL Data Warehouse uncovers its performance insights that cater to enterprises seeking robust analytics capabilities. The platform's integration with Microsoft's Azure ecosystem enhances data processing speed and efficiency, leveraging cloud resources for parallel query execution. Azure SQL Data Warehouse's intelligent caching mechanisms optimize query performance, reducing latency and enhancing user experience. However, users must consider workload distribution and resource allocation strategies to maximize Azure SQL Data Warehouse's performance benefits, aligning data processing requirements with underlying storage configurations.
Storage Options
Navigating Microsoft Azure SQL Data Warehouse's storage options reveals a spectrum of choices tailored to diverse data handling needs. The platform's tiered storage architecture enables cost-effective management of frequently accessed and archival data, optimizing resource utilization. Azure SQL Data Warehouse's support for hybrid data scenarios bridges on-premises and cloud storage integrations, fostering a seamless data environment. While these storage options offer scalability and flexibility, users must strategize data partitioning and indexing methodologies to enhance query performance and storage efficiency.
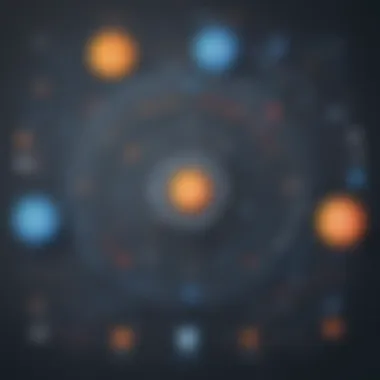
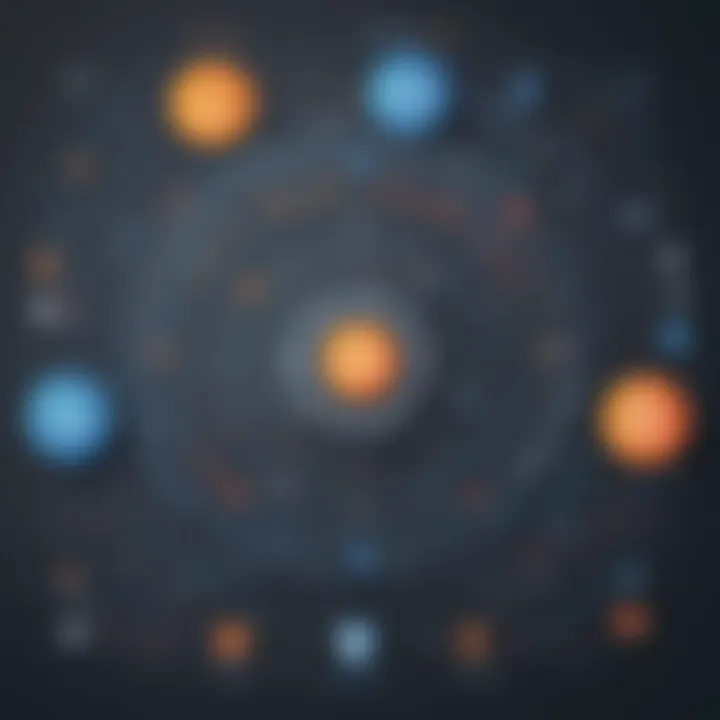
Implementation Considerations
Implementation considerations play a crucial role in the exploration of alternatives to BigQuery. In this article, we delve deep into the essential aspects that need to be addressed when considering a shift from BigQuery to other data storage and analytics solutions. By focusing on specific elements such as migration strategies, training, support, and performance optimization, businesses can ensure a seamless transition and efficient utilization of the chosen alternative.
Migration Strategies
Data Transfer Best Practices
Data transfer best practices are fundamental when aiming to migrate from BigQuery to a different data warehouse service. These practices entail efficient ways to move large volumes of data with minimal disruption to operations. By implementing best practices in data transfer, businesses can safeguard the integrity of their datasets and prevent data loss during the transition process. The strategic use of data transfer best practices can significantly contribute to a successful migration and ensure that the new data storage system operates optimally.
Downtime Mitigation
Downtime mitigation strategies are essential for minimizing business interruptions during the migration process. By employing effective downtime mitigation techniques, companies can reduce the impact of downtime on critical operations. Strategies such as scheduling migrations during off-peak hours and implementing failover mechanisms help mitigate the risk of prolonged downtimes. While downtime is inevitable during migration, efficient mitigation strategies can help companies manage disruptions effectively.
Training and Support
User Training Programs
User training programs are instrumental in preparing employees to work with the new data storage solution. These programs educate users on the functionalities, features, and best practices of the alternative to BigQuery. By providing comprehensive training, businesses can enhance user adoption rates, minimize errors, and increase productivity. User training programs are vital for empowering employees with the skills and knowledge needed to leverage the full potential of the selected data warehouse solution.
Vendor Support Evaluation
Evaluating vendor support services is crucial when selecting an alternative to BigQuery. Reliable vendor support ensures timely assistance in case of issues or technical challenges. By assessing the quality of vendor support, businesses can mitigate risks associated with downtime, data loss, or performance issues. A robust vendor support system is essential for maintaining system stability and resolving issues promptly.
Optimizing Performance
Tuning Query Execution
Optimizing query execution is key to improving the performance of a data warehouse solution. By tuning query execution, businesses can enhance query speed, reduce latency, and optimize resource utilization. Fine-tuning query execution involves optimizing query plans, indexing strategies, and data partitioning to achieve efficient query performance. Effective tuning of query execution is essential for maximizing the operational efficiency and responsiveness of the chosen data storage alternative.
Caching Strategies
Implementing caching strategies can significantly boost the performance of a data warehouse system. Caching allows frequently accessed data to be stored in memory, enabling rapid retrieval and reducing query processing time. By strategically implementing caching strategies, businesses can improve query performance, response times, and overall system efficiency. Caching strategies play a vital role in enhancing the data retrieval process and ensuring optimal performance of the selected data storage solution.
Conclusion
In this article, the significance of choosing the right alternative to BigQuery cannot be overstated. The careful consideration of various factors and requirements is crucial for small to medium-sized businesses, entrepreneurs, and IT professionals looking to optimize their data storage and analytics solutions. By exploring alternatives such as Snowflake, Amazon Redshift, Google Cloud Datastore, Apache Cassandra, and Microsoft Azure SQL Data Warehouse, decision-makers can make informed choices that align with their unique business goals and data security needs. The importance of concluding with a well-thought-out decision that balances needs and budget while future-proofing data warehouse solutions is paramount to long-term success in a competitive digital landscape.
Final Thoughts on Choosing Alternatives
Balancing Needs and Budget
Balancing needs and budget plays a pivotal role in selecting the optimal alternative to BigQuery. This aspect combines the necessity of meeting performance metrics, cost considerations, and scalability needs with the financial constraints of the business. By striking a delicate balance between these factors, organizations can ensure efficient operations while staying within budgetary confines. The key characteristic of balancing needs and budget lies in the ability to leverage cutting-edge data warehouse technologies without overspending, thus maximizing ROI. This approach proves advantageous for small to medium-sized businesses, entrepreneurs, and IT professionals seeking cost-effective yet powerful solutions. However, it is essential to acknowledge the potential trade-offs and limitations that come with prioritizing budget constraints, as this decision could impact long-term scalability and performance.