Unlocking the Potential: The Crucial Role of Data Lakes in SaaS Environments
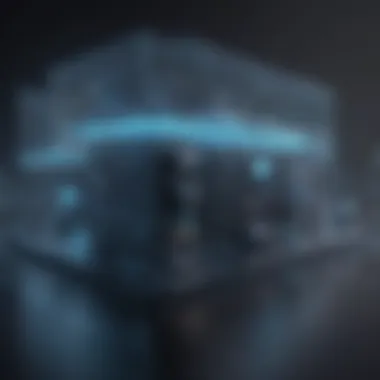
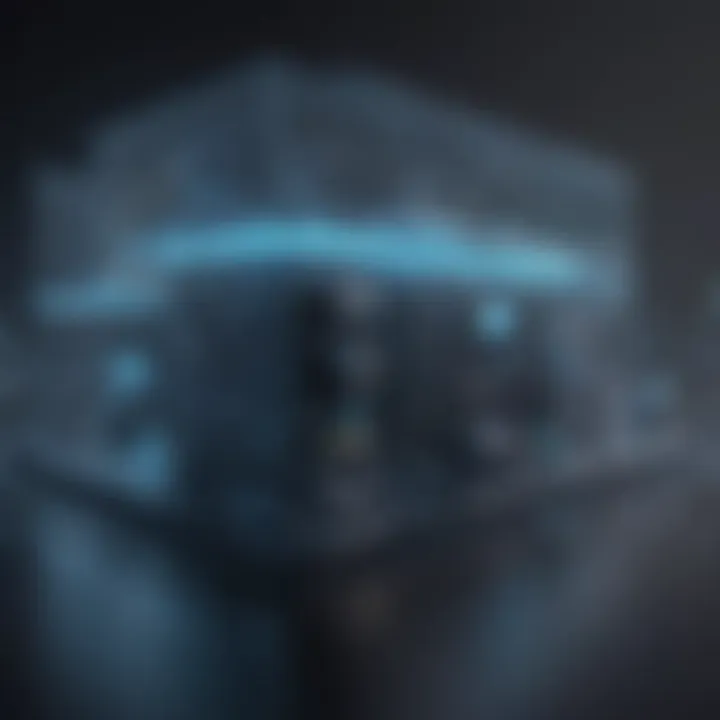
Software Insight
As we embark on an exploratory journey into the intertwined realms of Data Lake technology and Software as a Service (SaaS), it is crucial to lay a solid foundation by delving into how these two entities complement each other seamlessly. A Data Lake, a vast repository that can store structured and unstructured data in its native format until needed, plays a pivotal role in modern businesses embracing SaaS solutions to meet their ever-increasing data processing demands. Unveiling the intricate connections between Data Lakes and SaaS is imperative for comprehending the transformative impact they have on data management and analytics capabilities.
User Interface Odyssey
Traversing through the landscape of user experience within the realm of SaaS powered by Data Lakes, one can't overlook the significance of a user-friendly interface. Navigational elements that intuitively guide users through the software's functionalities are paramount for ensuring a seamless experience. Evaluating the learning curve is essential β a steep learning curve could hinder user adoption and efficiency. Furthermore, exploring customer support options and their reliability is crucial for guaranteeing that users receive prompt assistance when encountering challenges within the software ecosystem.
Performance and Stability Sojourn
As we delve into the performance and reliability facets of a SaaS platform integrated with Data Lake technology, the need for speed and operational efficiency cannot be overstated. Analyzing uptime and downtime statistics provides insights into the platform's stability and availability, critical factors for businesses reliant on uninterrupted data processing. Moreover, assessing the integration capabilities with other tools allows businesses to streamline their operations and enhance productivity by ensuring seamless workflows between interconnected software solutions.
Security and Compliance Expedition
The journey into the domain of security and compliance within the context of Data Lake-centric SaaS solutions unveils a realm governed by robust data encryption protocols and stringent security measures. Ensuring compliance with industry regulations is non-negotiable for businesses entrusted with sensitive data processing. Additionally, examining backup and disaster recovery measures safeguards businesses against potential data loss or system downtime, reinforcing the platform's reliability and data integrity.
Intro to Data Lake in SaaS
The realm of Software as a Service (SaaS) stands at the forefront of modern business operations, grappling with escalating data storage and processing demands. Amidst this landscape, the concept of Data Lake emerges as a pivotal asset, revolutionizing how businesses manage and harness their data resources. In this comprehensive guide, we delve into the integrative nature of Data Lake within the SaaS framework, shedding light on its significance in enhancing data management and analytics capabilities. Data Lake in SaaS represents a beacon of efficiency amidst the sea of data inundating modern businesses, offering a centralized repository for diverse data types and formats. Let's navigate through the depths of Data Lake in SaaS to appreciate its role in the digital transformation era.
Understanding Data Lakes
Conceptual Framework
A Conceptual Framework provides the theoretical underpinning for the design and implementation of Data Lakes in SaaS environments. It serves as a roadmap, defining the structure, relationships, and functionalities of Data Lakes within the broader context of SaaS applications. The versatility of a Conceptual Framework lies in its ability to adapt to varying business needs, ensuring a tailored approach to data management and analysis. While its adaptability is a hallmark strength, challenges may arise in aligning the framework with specific organizational requirements, necessitating diligent customization efforts to optimize its utility in a SaaS-driven ecosystem.
Key Components
Key Components within a Data Lake constitute the fundamental building blocks that enable efficient data storage, processing, and retrieval functionalities. From data ingestion mechanisms to storage layers, each component plays a crucial role in shaping the overall efficacy of a Data Lake in a SaaS context. The modular nature of Key Components allows for scalability and flexibility, catering to evolving data demands and technological advancements. However, striking a balance between performance and cost-efficiency remains a perpetual consideration in optimizing Key Components for seamless integration with SaaS platforms, warranting strategic decision-making and resource allocation.
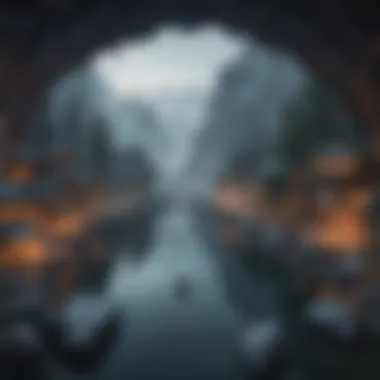
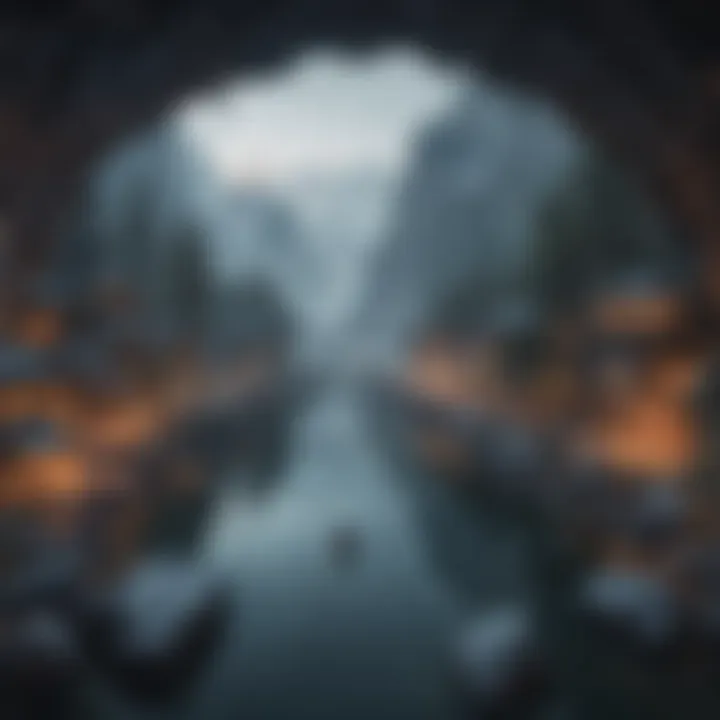
Evolution of SaaS
Origin Story
The Origin Story of SaaS traces back to a transformative period in software delivery, marked by a shift towards cloud-based services and subscription models. This paradigm shift heralded a new era of accessibility, affordability, and scalability in software provisioning, laying the foundation for the widespread adoption of SaaS solutions. The democratization of software access through the Origin Story of SaaS democratizes access to software applications previously confined to on-premises installations. However, challenges related to data security and compliance underscore the importance of robust governance frameworks and risk mitigation strategies to safeguard sensitive information in SaaS environments.
Market Dynamics
Market Dynamics in the SaaS sector highlight the interplay of supply and demand forces shaping industry trends, competitive landscapes, and consumer preferences. As SaaS offerings continue to proliferate across diverse sectors, market dynamics drive innovation, differentiation, and customer-centricity among service providers. Navigating the complex terrain of Market Dynamics equips businesses with insights into market trends, competitive threats, and growth opportunities, guiding strategic decision-making and resource allocation in an increasingly competitive SaaS landscape. Stay tuned as we explore the nuances of Data Lake integration with SaaS platforms and the transformative potential it holds for modern businesses engaging with cutting-edge data technologies.
Data Lake Architecture
In this part of our comprehensive guide on the role of Data Lake in SaaS, we delve into the essence of Data Lake Architecture. Data Lake Architecture plays a pivotal role in handling the vast and diverse data requirements of modern businesses. It serves as the foundational structure that enables efficient storage, management, and analysis of tremendous amounts of data. The architecture comprises various components that work in unison to ensure data accessibility, scalability, and reliability. By understanding Data Lake Architecture, businesses can optimize their data workflows, streamline processes, and derive valuable insights to drive strategic decisions and improve overall operational efficiency.
Batch Processing
Data Ingestion
When we discuss Data Ingestion within the context of Data Lake Architecture, we are focusing on the critical process of collecting, transferring, and loading data into the data lake system. Data Ingestion plays a crucial role in ensuring that data from diverse sources is efficiently imported into the data lake, facilitating unified storage and analysis. Its key characteristic lies in its ability to handle large volumes of data efficiently, enabling businesses to process and analyze vast datasets without complications. Data Ingestion stands out as a popular choice for this article due to its capability to streamline the data integration process, ensuring that relevant information is promptly available for analysis. While Data Ingestion simplifies the data flow process, businesses must consider factors like data quality, transformation requirements, and data compatibility to fully leverage its advantages within their data lake environment.
Storage Layers
Another fundamental aspect of Data Lake Architecture is Storage Layers, which define how data is stored within the data lake environment. These layers are designed to organize and categorize data based on factors like access frequency, data type, and performance requirements. The key characteristic of Storage Layers is their ability to manage data efficiently, ensuring that information is stored in a structured manner for easy retrieval and analysis. This choice is beneficial for this article as it emphasizes data organization and accessibility, enabling businesses to effectively manage and utilize their data resources. However, businesses should be mindful of potential challenges such as data duplication, storage costs, and scalability limitations when implementing Storage Layers in their data lake architecture.
Real-time Analytics
Stream Processing
In the realm of Data Lake Architecture, Stream Processing plays a pivotal role in enabling real-time analytics capabilities for businesses. Stream Processing focuses on the continuous processing of data streams as they are generated, allowing organizations to extract insights and take immediate actions based on up-to-date information. Its key characteristic lies in its ability to process data swiftly, providing businesses with the opportunity to make informed decisions in real time. Stream Processing is a beneficial choice for this article as it underscores the importance of leveraging data promptly for critical decision-making processes. By utilizing Stream Processing, businesses can harness the power of real-time data analytics, gaining a competitive edge in today's fast-paced business landscape. However, businesses need to consider factors like data latency, processing complexity, and system resources to effectively leverage Stream Processing in their data lake architecture.
Complex Event Processing
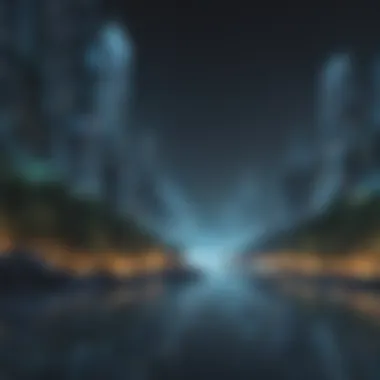
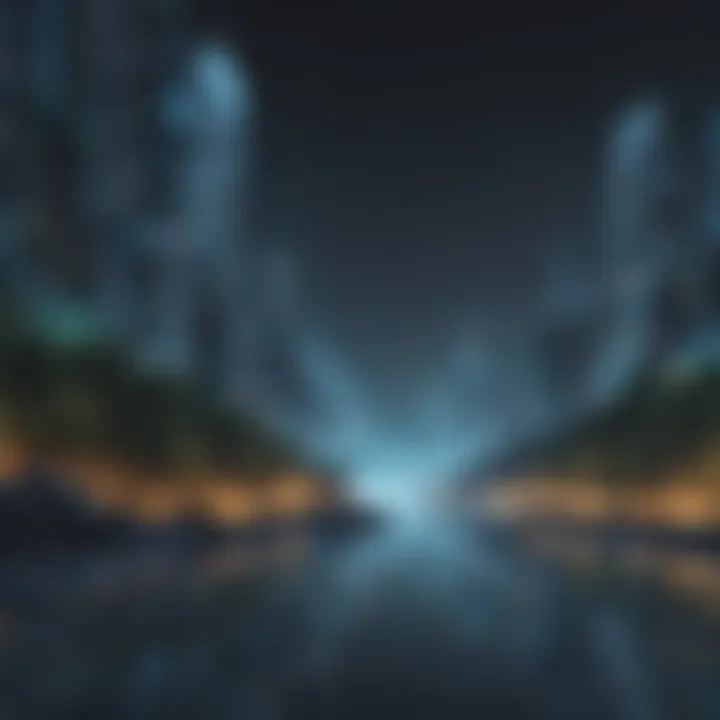
Complex Event Processing (CEP) stands out as another crucial component of Data Lake Architecture, focusing on the analysis of patterns and relationships within data streams to identify significant events or trends. CEP's key characteristic lies in its ability to detect complex patterns in real time, enabling businesses to detect anomalies, trends, and opportunities promptly. This choice is beneficial for this article as it highlights the sophisticated analytical capabilities of CEP in enhancing data insights and decision-making processes. By embracing Complex Event Processing, businesses can uncover valuable insights from their data streams, enabling proactive responses to emerging trends and events. However, businesses must address challenges such as event correlation, data accuracy, and rule complexity when implementing CEP within their data lake environment.
Integration of Data Lake with SaaS
In the intricate landscape of Software as a Service (SaaS), the integration of Data Lake stands as a cornerstone of strategic decision-making. This section delves into the crucial link between Data Lake and SaaS, shedding light on how this amalgamation revolutionizes data management and analytics. By intertwining the vast storage capabilities of Data Lake with the dynamic functionalities of SaaS, businesses pave the way for enhanced efficiencies and unprecedented insights. This integration serves as the bedrock for scalable data solutions and informed decision-making processes.
Advantages of Integration
Enhanced Scalability
The concept of Enhanced Scalability offers a paradigm shift in the world of data management within SaaS environments. By leveraging Enhanced Scalability, organizations can effortlessly adapt to fluctuating data volumes and processing demands without compromising operational efficiency. The key characteristic of Enhanced Scalability lies in its ability to expand or contract resources seamlessly, ensuring optimal performance under varying workloads. This flexibility not only boosts productivity but also minimizes resource wastage, making it a preferred choice for businesses seeking sustainable growth.
Improved Data Insights
Improved Data Insights serve as the guiding beacon for informed decision-making and strategic planning within SaaS implementations. This facet accentuates the importance of leveraging data to extract actionable insights, driving business success and competitive advantage. The hallmark of Improved Data Insights lies in its capacity to transform raw data into meaningful information, offering invaluable perspectives for business leaders. By harnessing this capability, organizations can uncover hidden trends, enhance operational efficiencies, and unlock new revenue streams, positioning Improved Data Insights as an indispensable asset in the realm of data analytics.
Challenges Faced
Data Security
Preserving the sanctity of data holds paramount importance in the era of advanced analytics and digital transformation. Data Security emerges as a critical aspect of the integration between Data Lake and SaaS, safeguarding sensitive information from unauthorized access or breaches. The primary characteristic of Data Security lies in its ability to establish robust protocols and encryption mechanisms to prevent data vulnerabilities and ensure compliance with stringent data protection regulations. While Data Security fortifies data integrity, it also necessitates continuous vigilance and proactive measures to mitigate evolving cyber threats, underscoring its significance in data governance strategies.
Interoperability Issues
Navigating the complexities of interoperability stands as a formidable challenge in the seamless integration of Data Lake with SaaS platforms. Interoperability Issues encompass a range of technical obstacles, encompassing data format inconsistencies, protocol mismatches, and system compatibility constraints. The key characteristic of Interoperability Issues centers on the interoperable exchange of data between disparate systems, requiring strategic solutions to streamline data flow and eliminate integration silos. Despite its benefits in enabling cross-platform functionality, Interoperability Issues demand meticulous planning and agile responses to ensure data harmonization and operational cohesion.
This insightful analysis illuminates the multidimensional facets of integrating Data Lake with SaaS, underscoring the transformative potential and inherent complexities within this symbiotic relationship.
Data Governance in SaaS Data Lakes
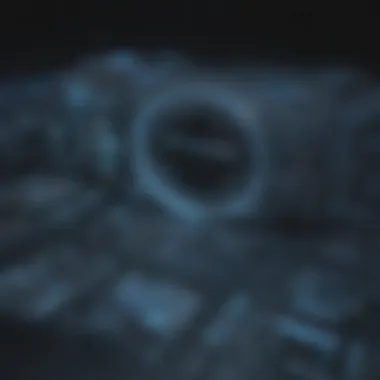
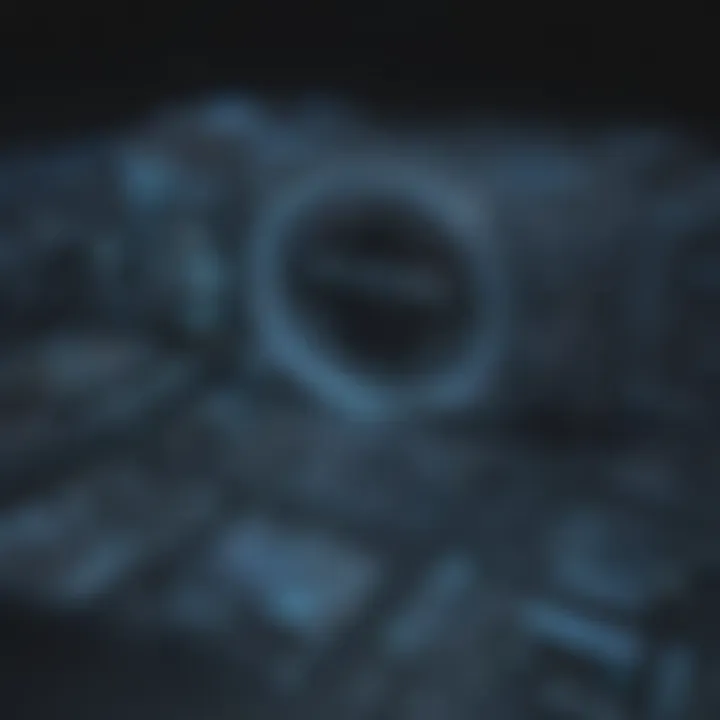
Data governance within Software as a Service (SaaS) data lakes holds a critical position in ensuring the effective management and utilization of data in the modern business landscape. As organizations grapple with escalating data volumes and diverse data sources, implementing robust data governance practices becomes imperative for maintaining data integrity, security, and compliance. In the context of this comprehensive guide on the role of data lakes in SaaS, the focus on data governance brings to light key elements that act as the foundation for successful data management strategies.
Role of Data Governance
Ensuring Compliance
Exploring the facet of ensuring compliance within data governance reveals its significant role in aligning organizational data practices with regulatory standards and requirements. By emphasizing compliance measures within SaaS data lakes, companies can mitigate the risks associated with data breaches, privacy violations, and non-compliance penalties. Ensuring compliance stands out as a cornerstone principle that upholds the ethical and legal obligations of organizations towards their data handling processes.
Maintaining Data Quality
The aspect of maintaining data quality under the umbrella of data governance underscores its essence in preserving the accuracy, consistency, and reliability of data assets. By focusing on data quality maintenance, businesses can enhance decision-making processes, foster trust in data-driven insights, and streamline operational efficiencies. The meticulous approach to data quality within SaaS data lakes contributes to establishing a solid data foundation that supports business growth and innovation.
Best Practices
Delving into best practices within data governance sheds light on optimal approaches for implementing and upholding data management standards within SaaS environments. Through effective data classification mechanisms, organizations can categorize data assets based on their sensitivity, importance, and regulatory requirements. This classification framework enables streamlined data access controls, privacy protocols, and data lifecycle management strategies to safeguard data integrity and confidentiality.
Data Classification
The practice of data classification involves categorizing data entities into distinct classes or categories based on predefined criteria such as sensitivity, criticality, or accessibility levels. With a robust data classification framework in place, businesses can prioritize data handling protocols, allocate resources efficiently, and streamline data analytics initiatives. Data classification emerges as a foundational practice that empowers organizations to manage data proliferation and complexity effectively.
Access Control
Access control mechanisms play a pivotal role in governing data accessibility and usage privileges within SaaS data lakes. By implementing stringent access controls, organizations can restrict unauthorized data access, prevent data leaks, and enforce compliance with data protection regulations. Access control measures form a vital component of data governance best practices, ensuring that data confidentiality and integrity are preserved throughout the data lifecycle.
This holistic exploration of data governance principles within SaaS data lakes offers a nuanced understanding of the pivotal role played by data governance in shaping data management strategies and fortifying organizational data assets against diverse risks and challenges.
Future Trends in Data Lakes and SaaS
In exploring the future trends in the symbiotic domains of Data Lakes and Software as a Service (SaaS), we delve into the cutting edge of technological advancements reshaping the digital landscape. Understanding the evolution of AI and machine learning integration in data lakes is paramount for businesses aiming to stay ahead in data analytics and decision-making processes. Leveraging AI algorithms and machine learning models offers unparalleled insights into complex data sets, enabling organizations to unlock hidden patterns and predictive analytics capabilities. The fusion of AI and machine learning with data lakes enhances data processing speed, accuracy, and efficiency, ushering in a new era of data-driven decision-making. Embracing these future trends empowers businesses to optimize operations, drive innovation, and gain a competitive edge in the dynamic SaaS landscape.
AI and Machine Learning Integration
The integration of Artificial Intelligence (AI) and Machine Learning (ML) in data lakes revolutionizes data processing and analysis methodologies. AI-powered algorithms enable data lakes to perform advanced analytics tasks efficiently, allowing businesses to extract valuable insights from massive datasets. Machine learning algorithms integrated with data lakes automate pattern recognition, anomaly detection, and predictive analytics, augmenting decision-making capabilities. By harnessing the synergy between AI, ML, and data lakes, organizations can streamline data processes, enhance personalization, and drive targeted marketing strategies. The integration of AI and machine learning presents opportunities for continuous innovation and operational excellence, positioning businesses at the forefront of data-driven competitiveness.
Data Monetization Strategies
Data monetization strategies in the realm of data lakes and SaaS offer organizations lucrative avenues to capitalize on their data assets. By leveraging data lakes as repositories of valuable information, businesses can extract, refine, and monetize data to generate new revenue streams. Establishing robust data monetization frameworks involves transforming raw data into strategic assets and insights that hold market value. Implementing data monetization strategies requires businesses to deploy advanced analytics tools, develop data-driven products or services, and establish data partnerships to expand market reach. Monetizing data through subscription-based models, targeted advertising, or data-as-a-service offerings enables businesses to monetize data assets effectively. Embracing data monetization strategies unlocks untapped revenue opportunities, enables market differentiation, and drives sustainable growth in the competitive SaaS landscape.